Jo Bovy
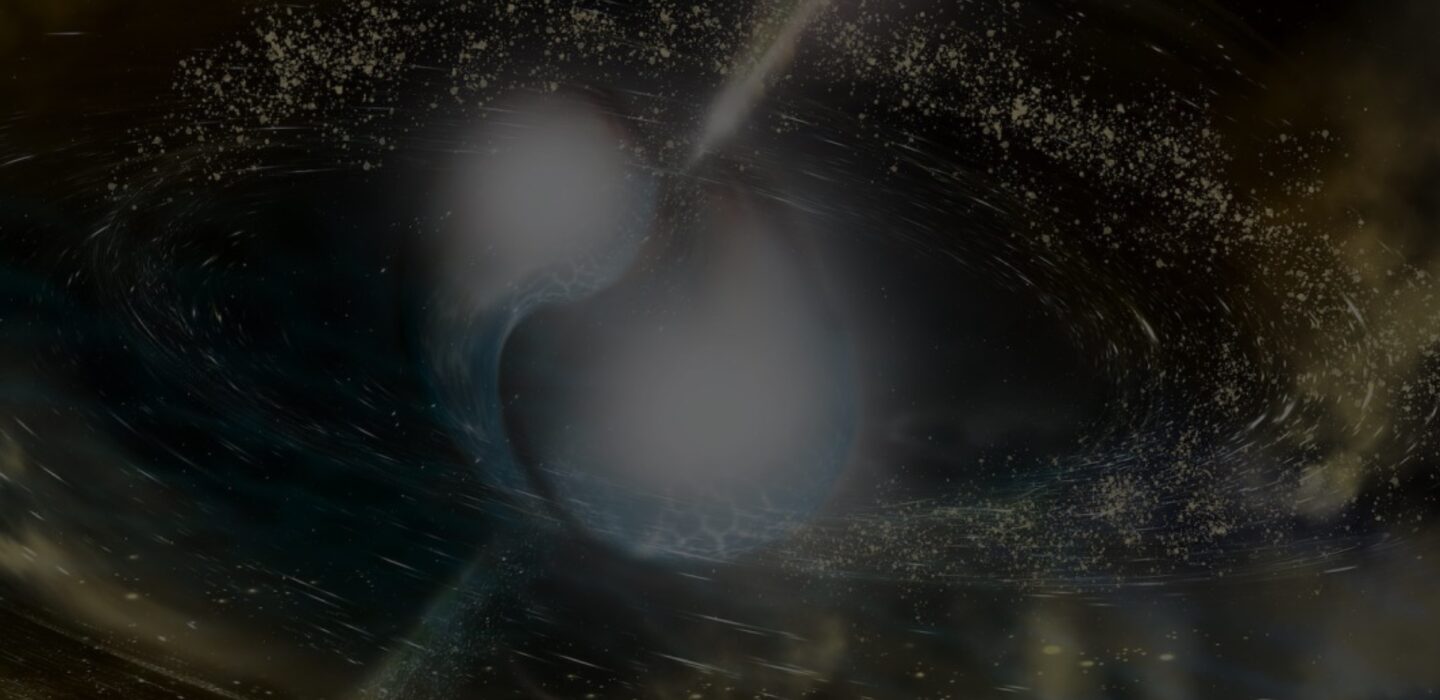
Tuesday, October 3 2024
4:00pm
Marlar lounge & via Zoom
Data-driven methods using a large variety of machine learning techniques have reshaped observational data analysis and data modelling in many fields of astrophysics in the last decade. However, despite their impressive performance, many of these methods struggle with issues of interpretability, selection biases, confounding factors, and ad hocness. In this talk, I will focus on these issues through the lens of my group’s work on large stellar survey databases such as Gaia and APOGEE. Finally, I will discuss our efforts towards building broader “foundation models” for astronomy: large models that can perform a large variety of machine-learning tasks traditionally performed by bespoke models and that can be adapted to future tasks without full re-training.